AI Adoption by Rural SMEs: Exploring Current Trends
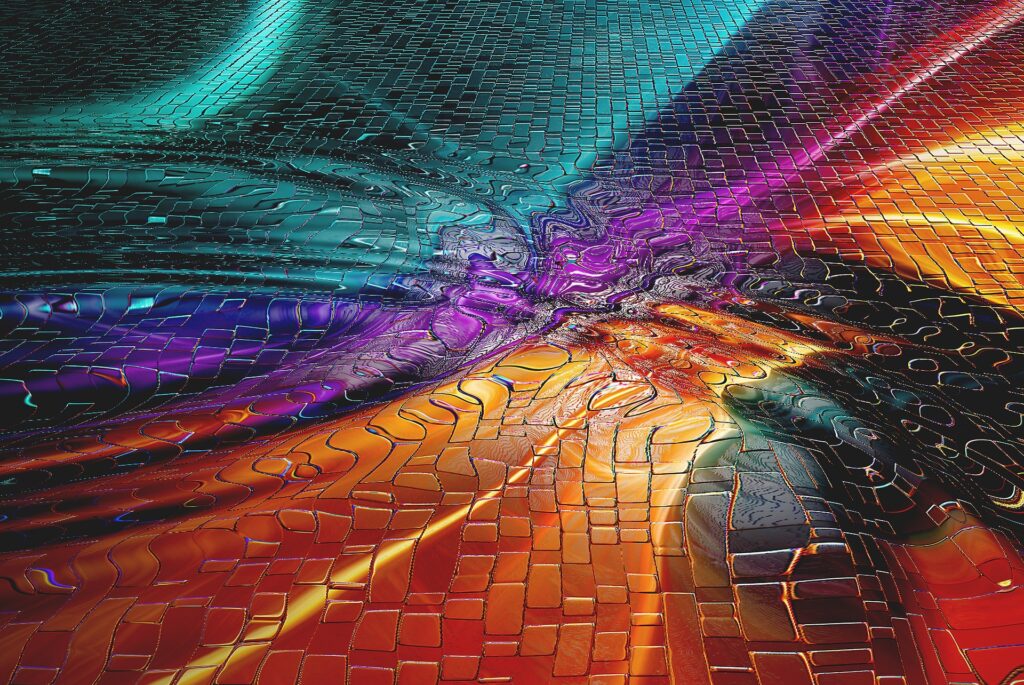
Dr David Dowell, Senior Lecturer in Marketing, University of St Andrews; Dr Wyn Morris, Senior Lecturer in Management, Aberystwyth University; Dr Robert Bowen, Lecturer in International Entrepreneurship, Cardiff University.
Introduction
This article debates the use of Artificial Intelligence (AI) in regional contexts, specifically rural small businesses (SMEs). AI could arguably play a leading role in the advancement of rural SMEs, with spin-off benefits for local economies and communities. Digital advances offer opportunities in regional UK contexts for SMEs (Bowen & Morris, 2019), and AI in particular can help remove inequality and offer economic opportunities (Korinek & Stiglitz, 2021). Thus, using data from the Longitudinal Survey for Small Business (LSBS), a large UK panel survey, we investigate the uptake of AI in rural areas. We begin by profiling the SMEs which have adopted AI, and then proceed to look for potential future directions of AI in rural SMEs. Before undertaking this data analysis, we review AI in rural business.
AI – Potential in Rural Areas?
AI has the ability to transform social and economic worlds, transforming the business world and entire sectors (Nishant et al., 2020). Traditionally, industrial progress drives urbanisation (Baráth & Futó, 1984), with regions and rural areas left much changed. Based on the historically unprecedented exponential growth of data, platform use and social media – AI is thriving (Lazzeretti et al., 2023), which, given its implications, poses different challenges and opportunities for rural areas given a new industrial age and innovation wave is upon us. Thus, several questions surrounding AI and rural areas are in need of debate.
The advent of AI may be seen as the latest stage in automation and mechanisation, or perhaps something more disruptive and the next step in innovation progress (Korinek & Stiglitz, 2019). Innovations may follow path dependence, where a region’s past predicts its future (EEG) or an alternative yet complementary new path with new assets and processes (Doloreux & Turkina, 2021). With respect to SMEs we may not have industrial clusters, nor sector dependence, therefore the history of a region may not be an apparent diver in AI adoption. However, past technology adoption, internet access and other variables may be associated with AI adoption in rural areas. This leads to RQ1: what is the profile of rural SME’s who are harnessing AI?
Korinek et al. (2021) argue that as opposed to prior revolutions, AI has a profound and perhaps the ultimate challenge to not just its influence on the type of work undertaken, the sectors, and the production methods, but rather to ‘employment’ more generally. It is further argued that AI may indeed do away with many forms of work and not offer alternatives, which have been part of prior mass changes (Anton et al., 2021). Hence, the second area of inquiry beyond who is adopting, is what their plans are for the future. RQ2: What are the future intentions of rural AI adopters?
Data and Analysis
This research uses data from the Longitudinal Small Business Survey (LSBS), which has been running since 2015, and at the time of publication, numerous waves of data collection have been completed. The focus is on small businesses, drawn from a variety of sectors. In 2022, participants were asked if they used AI (and other technologies). This was in addition to questions which were more general about the SME, such as its characteristics and ambitions.
Which SMEs are using AI?
There was n=9492 SMEs in the sample, n=3040 (7.10%) were classified as rural. The LSBS is designed to be representative of the UK Nations and based on the overall sample 77.43% were drawn from England, 9.51% Scotland, 6.95% Wales, 6.10% from Northen Ireland. Of the rural SMEs n=213 (7.01%) were ‘using’ AI, which was significantly lower than the urban part of the sample. The sectors that included rural AI users saw 45.07% in production and construction, 18.31% in transport, retail, food and accommodation services, 29.58% in business services, and 7.04% in other services.
Rural users of AI were more likely to have a business plan up to date (52.11%) than rural non-users of AI (28.72%). The rural SMEs who had adopted AI were mostly likely to have employees (86.38%), with a mean employment of 28.9 employees. The turnover for the rural AI users was £6,215712.30, well above the rural non-users (£2,265763.40). The rural AI users also have a high proportion engaged in exporting (43.66%), which is well above the rural non-users (17.47%). The percentage involved with innovation in the past three years was also much higher in urban businesses, 67.14% as opposed to 31.94% for rural users and non-users of AI.
Will they grow, export or expand?
Having profiled the SMEs in rural areas which use AI, we now focus on their future intentions in terms of AI adoption. By doing so, we can understand if there may be some positive future benefits generated by AI users. In terms of expected turnover in the coming financial year, 48.36% of the rural AI users were expecting and increased turnover, as opposed to the non-AI rural cohort, where only 33.96% expected an increase. In the same order, 38.50% and 49.52% expected turnover to remain the same as the previous year. For future plans beyond this[1] 76.47% of rural AI users wish to enhance their staff through skill development, more than the rural non-users 58.69%. Furthermore, 80.39% of rural AI users wished to increase their staff size within the UK, compared with 55.70% of non-AI rural SMEs. In terms of innovation, 66.67% planned to launch new offerings, 64.71% planned to invest in research and development, and in both cases, this was higher than the rural non-AI users. In terms of exporting, 39.22% of rural AI adopters were looking for enhanced exporting output, above the non-AI users (13.68%).
A notable side result is the intentions of SMEs around reducing carbon emissions and greenhouse gases. For the whole cohort sample (n=2387) 45.89% indicated that they agreed, and over the next three years would make attempts to reduce environmental damaging practices. Both the rural and urban AI users had higher proportions of agreement than the non-AI users. Furthermore, 58.82% of rural AI users were looking to decrease adverse environmental effects in the next three years.
Concluding Remarks
The industrial revolution drove high levels of urbanisation, and governments were forced to respond to mitigate negative effects (Anton et al., 2021). The AI revolution, as argued here, poses some challenges, but could be of ultimate benefit to rural areas, in particular through SME adoption and use. The profile of the current AI users suggests more engaged businesses, which tend to be larger, have higher levels of innovation and are more likely to be engaged in exports. These businesses may be considered as engaged and active; however case studies are needed around their adoption, and use of AI to help inform other SMEs and policy makers.
The second part of this research insight was to understand the future intentions of rural SMEs who are currently using AI. The benefits of their plans could, if realised, lead to spillovers, and benefits for rural areas. Growth, expansion, innovation and even sustainability are all more prominent plans amongst rural AI adopters than rural non-adopters of AI. Thus, if these businesses realise their plans the AI revolution may have serious positive implications for rural areas.
By understanding this, we can as a byproduct understand which SMEs are not using AI technologies and target them with policy. Yet the decision to target needs to be premised on the benefits which AI users intend to bring to rural areas, thus our second research question.
References
Data Source:
Department for Business, Energy and Industrial Strategy. (2024). Longitudinal Small Business Survey, 2015-2022: Secure Access. [data collection]. 8th Edition. UK Data Service. SN: 8261, DOI: http://doi.org/10.5255/UKDA-SN-8261-8
Literature:
Anton, K., Martin, S., & Joseph, S. (2021). Technological Progress, Artificial Intelligence, and Inclusive Growth. International Monetary Fund.
Baráth, E., & Futó, I. (1984). A Regional Planning System Based on Artificial Intelligence Concepts. Papers in Regional Science, 55(1), 135–154. https://doi.org/10.1111/j.1435-5597.1984.tb00832.x
Bowen, R., & Morris, W. (2019). The digital divide: Implications for agribusiness and entrepreneurship. Lessons from Wales. Journal of Rural Studies, 72, 75–84. https://doi.org/10.1016/j.jrurstud.2019.10.031
Doloreux, D., & Turkina, E. (2021). New path creation in the artificial intelligence industry: Regional preconditions, new actors and their collective actions, and policies. Regional Studies, 55(10–11), 1751–1763. https://doi.org/10.1080/00343404.2021.1919610
Korinek, A., & Stiglitz, J. E. (2019). 14. Artificial Intelligence and Its Implications for Income Distribution and Unemployment. In 14. Artificial Intelligence and Its Implications for Income Distribution and Unemployment (pp. 349–390). University of Chicago Press. https://doi.org/10.7208/9780226613475-016
Korinek, A., & Stiglitz, J. E. (2021). Artificial Intelligence, Globalization, and Strategies for Economic Development (Working Paper 28453). National Bureau of Economic Research. https://doi.org/10.3386/w28453
Lazzeretti, L., Innocenti, N., Nannelli, M., & Oliva, S. (2023). The emergence of artificial intelligence in the regional sciences: A literature review. European Planning Studies, 31(7), 1304–1324. https://doi.org/10.1080/09654313.2022.2101880
Nishant, R., Kennedy, M., & Corbett, J. (2020). Artificial intelligence for sustainability: Challenges, opportunities, and a research agenda. International Journal of Information Management, 53, 102104. https://doi.org/10.1016/j.ijinfomgt.2020.102104
[1] The LSBS sample includes internal cohorts, and for some parts of the questionnaire only one cohort will answer the questions. For future SME plans n=2399, rural n=753, rural AI users n=51.